In 2003/04, Arsene Wenger's Arsenal made history becoming the only team in Premier League history to have gone the full 38 games undefeated - a feat that has not since been replicated, with Preston North End the only other team to also go a whole season unbeaten in the domestic top flight, back in 1889!
Statsbomb's free data repository now includes all of Arsenal's games for that season. Using density plots in R and by dividing up the pitch areas into 'bins', we can show where certain elements of the game occurred. As we are dealing with 38 games worth of data, it is often quite cluttered thus hard to obtain anything useful from a graph which includes each individual data point, as shown below.
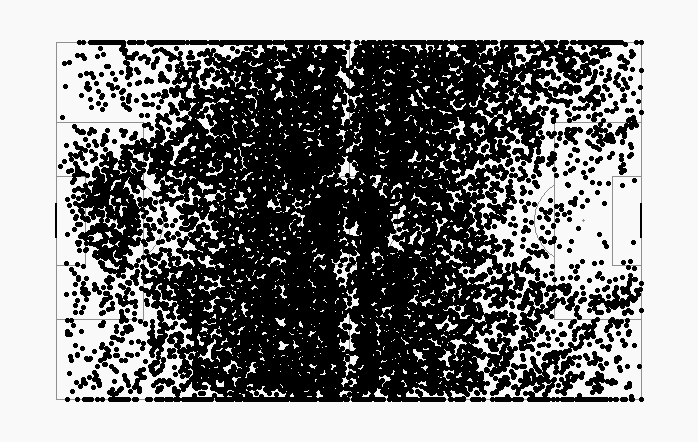
This shows the pass locations of every pass that the Invincibles played during the season. There are far too many points to obtain any useful information, we could filter down to look at specific instances, such as only those unsuccessfully played in the final third:
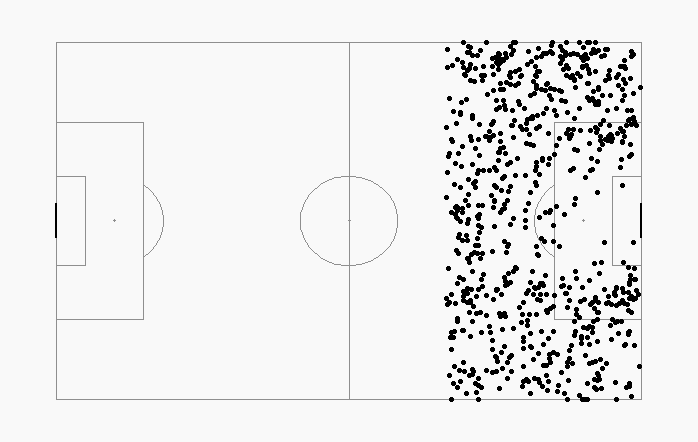
But even this gives us limited information, and makes it extremely difficult to make any serious conclusions. This is why heat maps are instead used. They can show the density/the amount of times that an event occurs in that area of the pitch. Here, we see all completed passes for the Invincibles.
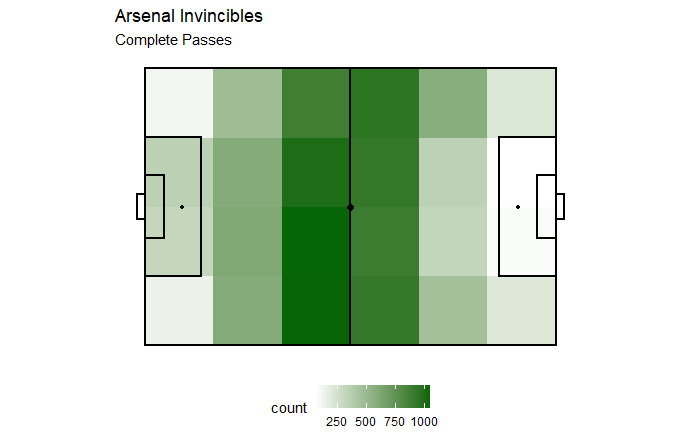
This plot is much more useful in showing us the balance of a team, and as we know that the Invincibles were a title winning side, playing with the time's traditional 4-4-2 (but with Bergkamp often sitting behind Henry similarly to the modern 4-2-3-1), it is unsurprising that there is a fairly equal distribution of play down the right and left flanks. They were not overly reliant on any one player - an excellent squad. Unfortunately, the Premier League does not have advanced statistics going back this far on their website, but it is fair to say that they would have held lots of possession and built up from the back, as was synonymous with all of Wenger's Arsenal sides. Easy passes across the back four as part of the build up play would have accumulated over the season, showing why there is a greater density of passes in the middle third, as the opposition would have sat back in the hope of nullifying the Arsenal Greats.
Next, we can similarly look at the incompleted passes played. I have stuck to the intuitive thought train when it comes to colouring the plots - green for good, and red for bad. Both work from a white base to show a low total count, all the way up to a strong green/red for where the event occurs most often. Often this may be shown in the traditional colour spectrum heat map form, however I think that this makes more sense, and is easier for the eye to quantify value. If the event does not occur in that area of the pitch then why should it be coloured at all?
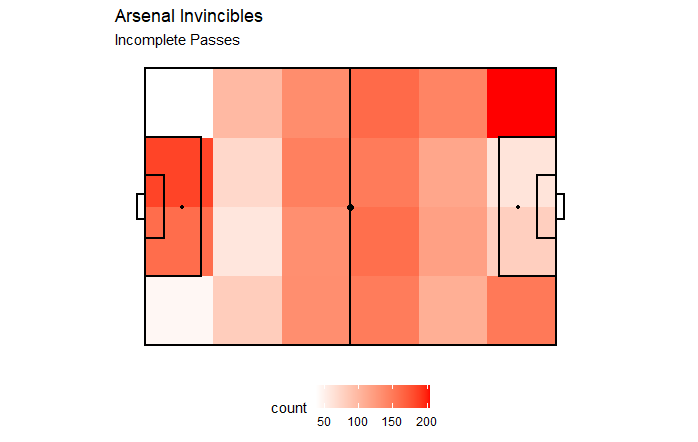
The first thing I would point out for this plot is the large change in scale, as you would expect with any football teams (even relegation stragglers), there are many more completed than incomplete passes. Next, the two areas that my mind notices are the left corner flag area, and Arsenal's own goal. Why are there so many misplaced passes in both these areas? At this point, our plot has successfully shown us a possible weakness in their team, and we could then delve further into finding out why this is. I would suspect it to be due to lost headers from corners and goal kicks. For now, as my focus is mainly on learning these plotting skills, I won't go into further detail, but it would be looking at the types of passes played from those areas and pressure on the ball, for example. This may reveal that the goalkeeper, Lehmann, often struggled to play out from the back, and this insight would be helpful for a team preparing for this opposition, as they may look to play with a high press.
Another great benefit of making these plots in R is that you can also separate it into several plots based on player, for example, to see how each individual holds up in each type of event. This insight could be used to coordinate a press, for example, if it seems that the left back struggles to play out from the back, then a team may want to put greater pressure on him each time he gets the ball to increase their likelihood of dispossessing the defender.
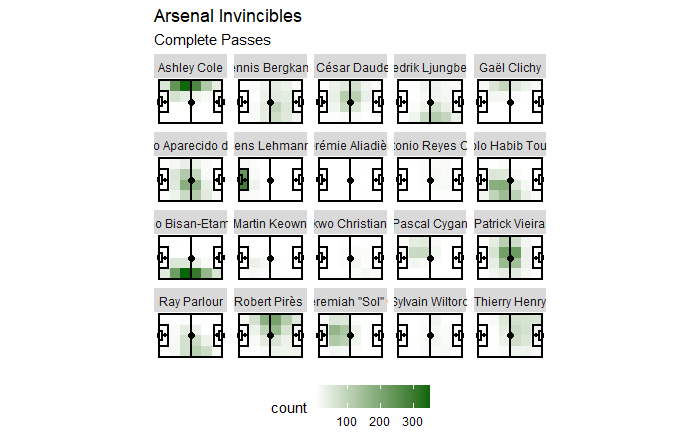
My first thought is that it would have been most useful to make all statistics per90. There is a clear bias towards players who featured more often, for example first choice left back Ashley Cole shows a much darker plot than his understudy Gael Clichy. Cole didn't necessarily have a better passing ability, but some might take that away from this plot. My second thought is that defenders often play much greater number of passes than attackers, as they have more time and less pressure, teams often play easy passes across the back. These easy passes won't hurt a team like those played in the final third, which require real guise and skill, however this plot may lead people to suspect that Kolo Toure was a better passer than the great Dennis Bergkamp. To improve this, we could factor in passes played under pressure only, and this should remove some of the bias. To maintain high professional standards, the plot should also be made larger so that full names can be seen for each player.
Next, to remove some of the bias based towards defenders I looked at positioning when receiving the football. This would bring up the numbers for attacking players who would often dribble or shoot as opposed to just passing the football. Now, we are really starting to see an individual's positioning and how it works within the team, however there is still an emphasis on the starting 11. As my focus was purely on building these types of plots and overcoming all of the challenges that it posed me, I did not go into great detail with correcting the statistics to per90, but this is definitely something that would be beneficial going forwards.
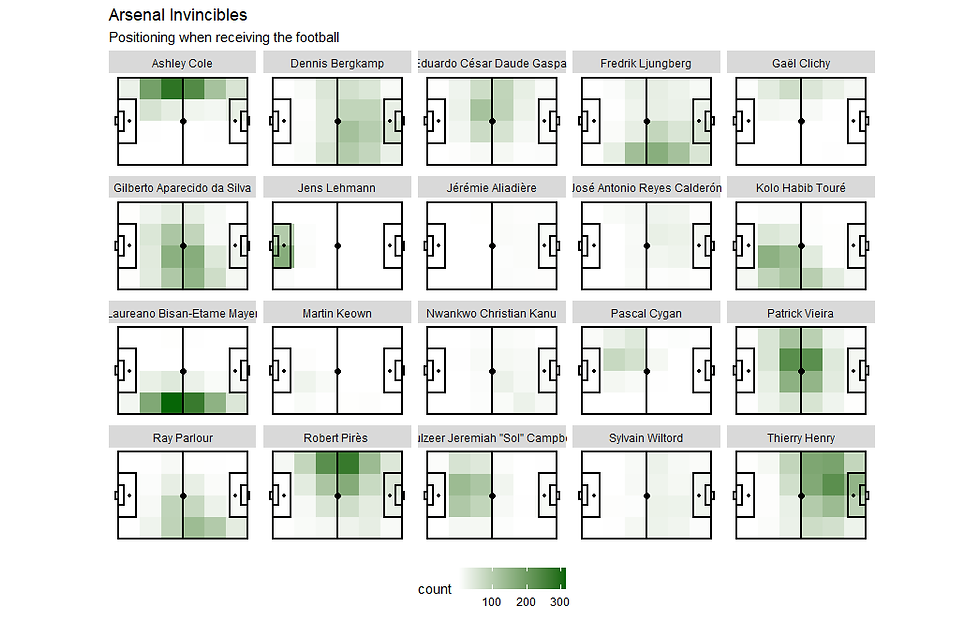
It is easy to see the normal starting 11 from this - Lehmann in goal, a back four of Cole, Campbell, Toure and Lauren, the middle of the park being controlled by Gilberto and Vieira, with Edu often featuring also, out wide was usually Pires on the left and Ljungberg on the right, with possibly the most dangerous front two in Premier League history - Bergkamp and Henry - sitting up top. We can also see how Player of the Season and Top Goalscorer (smashing in an unbelievable 30 goals) Theirry Henry was given the license to come away from the middle and receive the ball out on the left, allowing for him to drive at goal to create an opportunity for himself. Bergkamp also shows himself receiving the ball all over the park, showing how he was already resembling the number 10 in a 4-2-3-1 system before teams were even playing it. Henry had the pace to beat players in behind, so could hang off the last man's shoulder whilst Bergkamp tried to pull the strings from in between the lines.
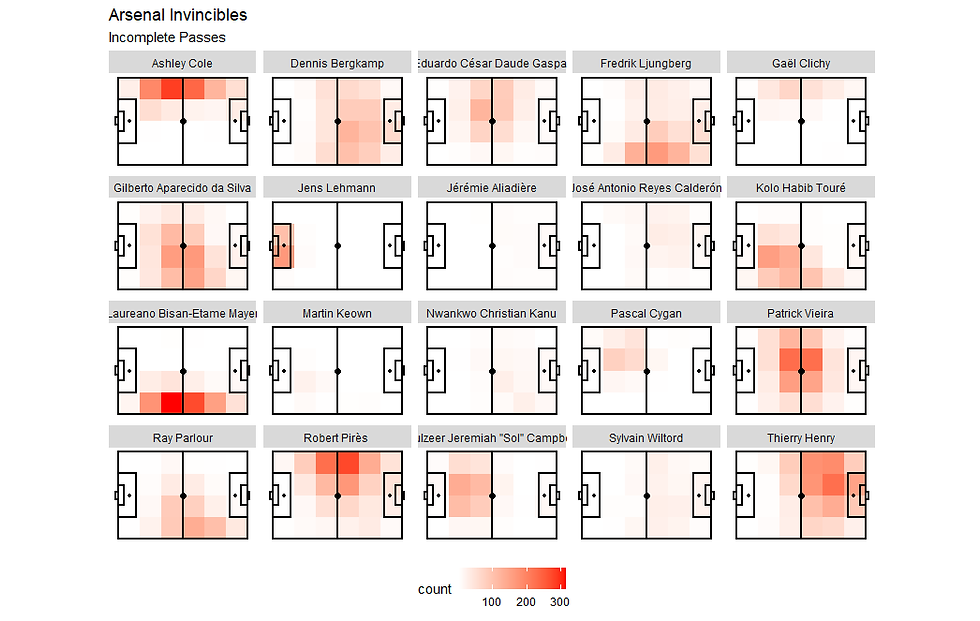
Moving on to look at missplaced passes for an individual again doesn't necessarily give us a great amount of insight. Even looking at it per90, it would still similarly show players losing the ball in their position and a fairly even distribution. Here, looking at passing success rates may give a better insight. This would also need to factor in a minimum number of passes for each 'bin' to prevent any larger or low scores confusing the plot's message. If they are unlikely to receive the ball in a certain area, then suggesting to put pressure on a player when they receive it there is futile.
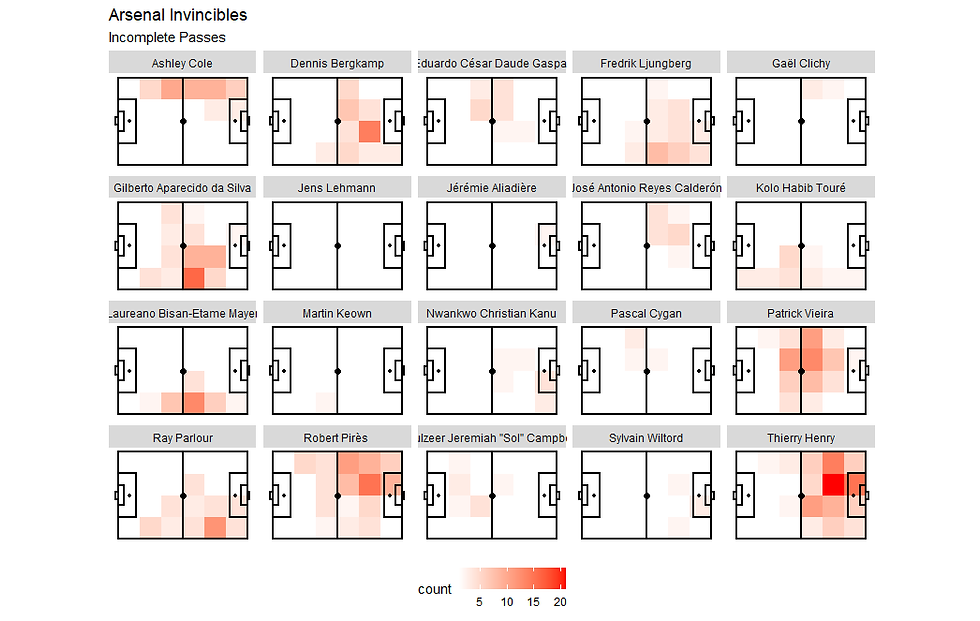
Once we filter the data for incomplete passes to only include those played along the ground we immediately get a different image. This takes away any long ball effect, so for example Lehmann goes from having quite a lot of missplaced passes from his penalty box to what looks like none across the entire season on this plot - this is beneficial for answering the previously asked question on if Lehmann should be put under pressure, as this plot suggests that he is in fact comfortable playing out from the back, or simply doesn't take the risk, with all his misplaced passes seeming to actually come from not winning long range goal kicks.
Using this format seems to remove the bias towards defenders also, with the ball being lost most often by more creative players further up the pitch. We now also see Pires likes to come off of his wing, with a lot of his missplaced passes in the same area as Henry's, just on the left of the opposition's 18 yard box. With Vieira playing directly behind these two, no doubt attempting to feed them both through into the final third, this plot could be useful for informing managers of the danger of Arsenal's inside left, and as a result one might possibly advise that the more defensive of the two centre midfielders (assuming the team plays the traditional 4-4-2 of the time) looks to cover this area, as opposed to more central areas, so that wide players can pass runners like Pires onto him, and hopefully stifle the Arsenal attack.
As well as dividing the plots up by players, it can also be done by team. This Statsbomb data only includes games that the Invincibles played in, so we are looking at both the home and away fixture that Arsenal played against each opposition. This can be done to assess how each team performed against Arsenal. In the below plot, it shows where Arsenal pressured each team.
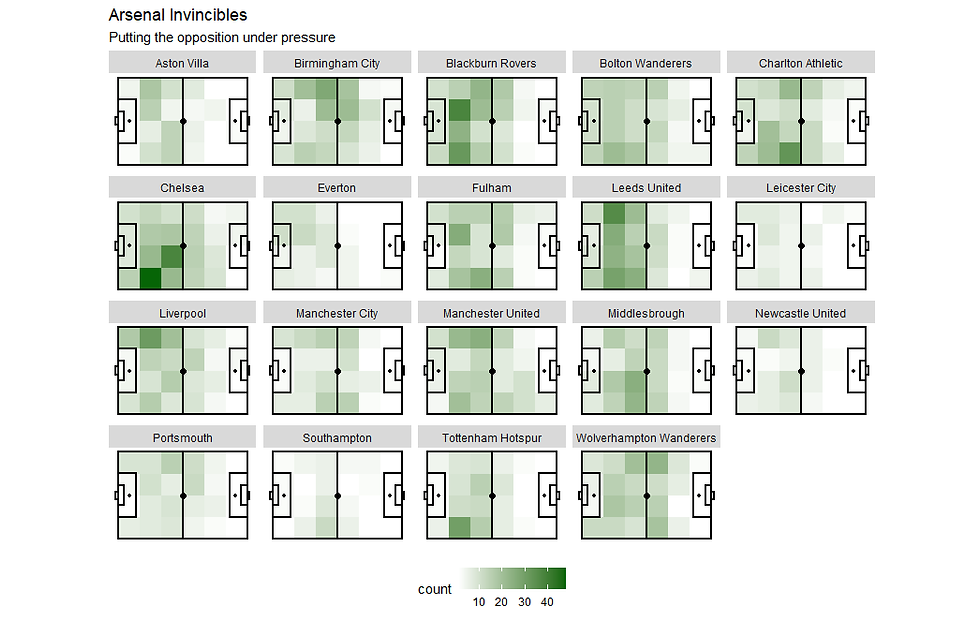
In this plot, it is worth remembering that more pressure isn't necessarily better. For example, the most pressure is put on against Chelsea, however they finished as Runners Up in the League, so it is unsurprising that they would have actually had a lot of the ball and thus Arsenal would have put more pressure on them in their games, even though Arsenal ran out 2-1 winners in both fixtures. Again, looking at Leicester, Arsenal put on barely any pressure, however Leicester finished 18th in the League, so were likely happy to sit back and try to play out for the point rather than taking the game to Arsenal. Ultimately, if this was the case, it worked as Leicester earned themselves a 1-1 draw whilst playing at home, however they still ended the season 6 points adrift of safety.
Arsenal are defending the leftmost goal in each pitch, so although it may be difficult to glean much from this plot without going into further detail, we can see that this team was not necessarily playing high pressing (or Gegenpress) football associated with some of today's more successful teams. However, they would also prefer to attempt to win the ball when its further from their goal, with large numbers of pressure coming out wide when they are more likely to be successful, by cutting off passing options for the opposition.
Overall, using these tiled heat maps is a good way of assessing events that occur frequently as it allows the plots to become less cluttered and easier for the eye to detect the important information. Whilst making the plots I had several issues, most commonly how to lay the football pitch lines onto the plot. Specifically, when using packages such as ggsoccer or statsbombR, I was able to create the pitch with a transparent fill so that the plot could be seen, however, the D at the 18 yard box would then become a full circle. In the end, I chose to draw my own pitch with a basic outline. Doing it this way meant that I have not included the D on the penalty box, or the circle on the half-way line. Although these are not essential and have no effect on the overall message being delivered, this would be the next step that I would take to further lift my professional standards.
As previously mentioned, when working with Big Data, it is important that we are drawing accurate conclusions and how we handle data is a big part of that. If I were doing analysis in a professional setting for a club or organisation, making statistics per90, or filtering data to get a better conclusion would be key to my work, however, for now when the main goal was to learn to produce these plots, I am happy to accept the way that they came out.
Another important factor to take this work further would be to include a direction of play arrow for Arsenal in each plot. Although we maybe assume that the attack is towards the right, this extra detail is key, especially when trying to explain what we have found to coaches, players and even more so for other key stakeholders who perhaps aren't as intrinsically involved in the game itself.
Finally, I think also including a value over the top of each tile could give the additional detail to someone immediately rather than them having to figure it out based on the intensity of the colour and where that marries up on the scale.
These are all additional thoughts that I have had during the making of these plots, and I will continue my learning and hope to add another post to my portfolio including these factors at a later date. Overall, I am pleased with the plots that I have produced, and ultimately the way that I persevered to overcome problems and found my own solutions rather than just copying code from someone else online. Hopefully, I can use these in the future to give valid insights into the way that a team plays, and any possible strengths or weaknesses that can be exposed and acted upon.
Comments